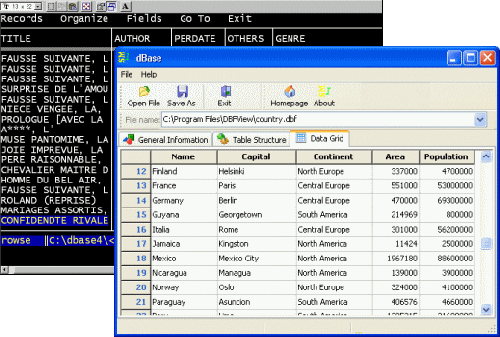
It just doesn’t seem like it was just 30 years ago that dBase came into our lives – WordStar, VisiCalc, and dBase on a PC with a green screen. In spite of their ubiquity now, the term "database" still hasn’t lost all of its magic. It holds out the promise of untold discovery – like when I googled "DBASE I" looking for a picture and found those two images on the first page of links [About 4,630,000 results (0.15 seconds)]. So when BRAINnet announces that they are making the "world’s largest brain database" available for free, it’s worth looking into. And it was. While I have some criticisms to make, including about their motives, I would still prefer their making it available to the alternative.
In a modern world where sophisticated queries, standardized relational design, and lightning fast search engines are the norm, the usefulness of a database is essentially a function of it’s contents. So let’s look at the contents of BRAINnet:
In large data arrays, multiple correlations are inevitable and our usual statistical methods become useless. While there are suggested correction methods, they are arbitrary and essentially untestable themselves. So a database like BRAINnet is likely to produce all sorts of correlations that people would love to say have predictive power [to sell as screening procedures]. That’s one of the big dangers of a huge database, and Brain Resource is right there in the middle of the soup – and it’s personalized medicine soup.
This is from Brain Resource‘s Integrative Platform for New Drug Discovery pdf. So one answer to the highlighted question is do a drug trial, but if the significance isn’t there in the study, go to the database and begin to run the numbers until you find a genotype in the group that does show a significant response. Voila`! A personalized anti-whatever for that genotype. It’s a two-fer. You have to be screened [$], and you buy the drug [$]. The variations on this theme are as numerous as the ripples in a stream, and the opportunities for the kind of science-lite we’ve seen in these last years is magnified geometrically. I’m in no position to accuse Brain Resource of that kind of sheenanigans [but Brain Resource‘s CEO and BRAINnet‘s CEO are both authors on those two papers up there, and the slide is from their own pdf].
Speaking of slides, this one is theirs too. Those logos look like the who’s who of the FDA’s Settlement Hall of Fame Fines. And the authors on their iSPOT study are from the Alumni Club of Senator Grassley’s Conflict of Interest Investigations [Williams LM, Rush AJ, Koslow SH, Wisniewski SR, Cooper NJ, Nemeroff CB, Schatzberg AF, Gordon E.]. Come to think of it, many of the gang of 44 from the Mayflower Action Group Initiative are equally suspect.
A pilot study
D. Spronk, M. Arns, K.J. Barnett, N.J. Cooper, E. Gordon
Journal of Affective Disorders 128 (2011) 41–48.
The aim of this study was to investigate if biomarkers in EEG, genetic and neuropsychological measures are suitable for the prediction of antidepressant treatment outcome in depression. Twenty-five patients diagnosed with major depressive disorder were assessed twice, pretreatment and at 8-wk follow-up, on a variety of QEEG and neuropsychological tasks. Additionally, cheek swab samples were collected to assess genetic predictors of treatment outcome. The primary outcome measure was the absolute decrease on the HAM-D rating scale. Regression models were built in order to investigate which markers contribute most to the decrease in absolute HAM-D scores. Patients who had a better clinical outcome were characterized by a decrease in the amplitude of the Auditory Oddball N1 at baseline. The ‘Met/Met’ variant of the COMT gene was the best genetic predictor of treatment outcome. Impaired verbal memory performance was the best cognitive predictor. Raised frontal Theta power was the best EEG predictor of change in HAM-D scores. A tentative integrative model showed that a combination of N1 amplitude at Pz and verbal memory performance accounted for the largest part of the explained variance. These markers may serve as new biomarkers suitable for the prediction of antidepressant treatment outcome.
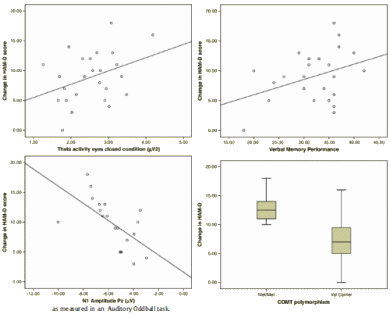
Well, it already looks like enthusiasm for genomic analysis might be waning:
http://www.nature.com/mp/journal/v16/n5/full/mp201038a.html
Review of 78 papers, 57 genes, 92 SNPs. Only four genes had reproducible results, but even they “may well be false positives,” per the authors.
Back to the drawing board!