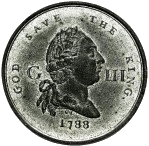
-
Gibbons RD, Hur K, Bhaumik DK, Mann JJ.Arch Gen Psychiatry. 2005 Feb;62(2):165-72.
-
Gibbons RD, Hur K, Bhaumik DK, Mann JJ.Am J Psychiatry. 2006 Nov;163(11):1898-904.
-
Nakagawa A, Grunebaum MF, Ellis SP, Oquendo MA, Kashima H, Gibbons RD, Mann JJ.J Clin Psychiatry. 2007 Jun;68(6):908-16.
-
Gibbons RD, Brown CH, Hur K, Marcus SM, Bhaumik DK, Mann JJ.Am J Psychiatry. 2007 Jul;164(7):1044-9.
-
Early evidence on the effects of regulators’ suicidality warnings on SSRI prescriptions and suicide in children and adolescents. [see peaks and valleys…]Gibbons RD, Brown CH, Hur K, Marcus SM, Bhaumik DK, Erkens JA, Herings RM, Mann JJ.Am J Psychiatry. 2007 Sep;164(9):1356-63.
-
Brown CH, Wyman PA, Brinales JM, Gibbons RD.Int Rev Psychiatry. 2007 Dec;19(6):617-31.
-
Gibbons RD, Segawa E, Karabatsos G, Amatya AK, Bhaumik DK, Brown CH, Kapur K, Marcus SM, Hur K, Mann JJ.Stat Med. 2008 May 20;27(11):1814-33.
-
Gibbons RD, Mann JJ.Drug Saf. 2011 May 1;34(5):375-95.
-
Reanalysis of the Randomized Placebo-Controlled Studies of Fluoxetine and VenlafaxineRobert D. Gibbons, C. Hendricks Brown, Kwan Hur, John M. Davis, and J. John MannArchives of General Psychiatry. Online February 6, 2012. [full text on-line]
-
Synthesis of 6-Week Patient-Level Outcomes From Double-blind Placebo-Controlled Randomized Trials of Fluoxetine and VenlafaxineRobert D. Gibbons, Kwan Hur, C. Hendricks Brown, John M. Davis, and J. John MannArchives of General Psychiatry. Online March 5, 2012.
-
Gibbons RD, Coca Perraillon M, Hur K, Conti RM, Valuck RJ, and Brent DAPharmacoepidemiologic Drug Safety. 2014 Sep 29. doi: 10.1002/pds.3713. [Epub ahead of print]
by Gibbons RD, Coca Perraillon M, Hur K, Conti RM, Valuck RJ, and Brent DAPharmacoepidemiologic Drug Safety. 2014 Sep 29. doi: 10.1002/pds.3713. [Epub ahead of print]
PURPOSE: In the 2004, FDA placed a black box warning on antidepressants for risk of suicidal thoughts and behavior in children and adolescents. The purpose of this paper is to examine the risk of suicide attempt and self-inflicted injury in depressed children ages 5-17 treated with antidepressants in two large observational datasets taking account time-varying confounding.METHODS: We analyzed two large US medical claims databases (MarketScan and LifeLink) containing 221,028 youth (ages 5-17) with new episodes of depression, with and without antidepressant treatment during the period of 2004-2009. Subjects were followed for up to 180 days. Marginal structural models were used to adjust for time-dependent confounding.RESULTS: For both datasets, significantly increased risk of suicide attempts and self-inflicted injury were seen during antidepressant treatment episodes in the unadjusted and simple covariate adjusted analyses. Marginal structural models revealed that the majority of the association is produced by dynamic confounding in the treatment selection process; estimated odds ratios were close to 1.0 consistent with the unadjusted and simple covariate adjusted association being a product of chance alone.CONCLUSIONS: Our analysis suggests antidepressant treatment selection is a product of both static and dynamic patient characteristics. Lack of adjustment for treatment selection based on dynamic patient characteristics can lead to the appearance of an association between antidepressant treatment and suicide attempts and self-inflicted injury among youths in unadjusted and simple covariate adjusted analyses. Marginal structural models can be used to adjust for static and dynamic treatment selection processes such as that likely encountered in observational studies of associations between antidepressant treatment selection, suicide and related behaviors in youth.
The statistical analysis was comprised of two stages. In the first stage, a logistic regression model was used to predict antidepressant usage on each of the 6months conditional on fixed covariates (demographics and prior suicide attempt and self-inflicted injury) and time-varying covariates (comorbid conditions, concomitant medications (listed above), psychiatric hospitalizations and psychotherapy above). The predicted probability of treatment at time point t was computed as the continued product of probabilities from baseline to time point t . The inverses of these estimated probabilities were then used as weights W(t) in the second stage analysis that related actual treatment (dynamcally determined on a month by month basis) to suicide attempt and self-inflicted injury using a discrete time survival model. In practice, W(t) is highly variable and fails to be normally distributed. To overcome this problem, Robins suggested use of the stabilized weight:where L is the set of all baseline and time-varying covariates, V is a subset of L consisting of only the baseline covariates (i.e. time invariant effects), A(k) is the actual treatment assignment at time k , and à (k) is the treatment history…
The paper is based on the analysis of two large longitudinal claims databases from which they extracted a number of covariates. He describes, but does not show, his analyses which I couldn’t exactly follow, but he could disappear the correlation between SSRIs and suicidality by his factor analysis. And like many of his papers, there’s nothing to say [because there’s nothing to see]. And like so much of his work, in spite of all the jargon, the only way there is to accept his conclusions is to take them on faith. I’m not willing to do that based on vetting his previous work [cataloged above]. As Neuroskeptic tweeted:
![]() |
the thing is, I might be willing to buy Gibbons et al’s argument about confounding, *but* I just can’t trust… |
![]() |
…him to present an unbiased analysis of the data, judging by what I’ve seen of the "CAT"…. |
[see Can A Computer Measure Your Mood? (CAT Part 3)] |
Well I certainly agree, but my skepticism goes further – beyond his CAT work, and the conduct of many of the studies on the list. Besides his practiced opaqueness and monotonous conclusions, I doubt that any population study of the problem of Akathisia and suicidality will ever shed any meaningful light on this question based on clinical experience. I’ve seen cases of agitation, and suicidality, and know of several related completed suicides. The most common version is a patient who gets put on an SSRI and becomes agitated, aggressive and they stop taking it either themselves or at the request of their parents – and they never go back to see the person that prescribed it. So they wouldn’t show up at all in a longitudinal population database. If you’re a clinician and you’ve seen these cases, even though they’re infrequent, you have no questions about the syndrome. It’s not subtle. These population studies use suicidality and completed suicides as an end-point rather that the full range of the presentations of Akathisia.
ACKNOWLEDGEMENTS This work was supported by NIMH grant MH8012201 (RDG) and AHRQ grants 7U19HS021093-03 (RDG) and T32HS000084 (MCP)… Dr. Gibbons has been an expert witness in suicide cases for the US Department of Justice, Wyeth and Pfizer.
The fine print in this report tells us that it is a climb down for Gibbons from his former denial. Now he does find a significant increase of suicidal behavior and attempts – in 2 independent data bases, no less! That is the ecologically important part of the report.
The rest of his paper is an effort to obfuscate the primary result by voodoo statistical hand waving. This attempt fails because he gives no guidance to clinicians about how to get their minds around the 24 ‘dynamic predictor’ variables in order to choose wisely for an individual patient. And, as Neuroskeptic points out above, this is the same guy who wants us to believe he can diagnose depression with just about 4-6 questions in an on-line rating scale – for a fee, of course.
Excellent point!
More of his “starts with a conclusion, and he just plugs away at trying to find a way to reach it” motif
Starting with a conclusion and making the numbers work was what I was taught in business school. Seems Dr. Gibbons has done rather well financially using this model.
Steve Lucas
Dr. Mickey, why not correspond with Dr. Gibbons and request his calculations?
I’m increasingly suspicious of these large population “studies” using insurance claim databases. First of all, the databases are open only to those (like Gibbons, and the authors of that lamentable BMJ “study” of youth suicide attempts) who are invited by the insurance company, and so are utterly un-checkable. Second, their coding often reveals little about the actual course of events and is vulnerable to the most manipulative mis-interpretation.
Third and maybe most worrisome, look who’s pushing it! This July news piece from NPR, there’s a major FDA initiative to increase this type of research, which comes with substantial private funding … from Pharma.
http://www.npr.org/blogs/health/2014/07/21/332290342/big-data-peeps-at-your-medical-records-to-find-drug-problems
This new system also operates to shield the “researchers” from any need to disclose their Pharma ties. Heaven forbid! They now need only say they’re funded by the Kaiser Fdn, or Harvard Pilgrim Health … or the NIH. Nice trick.